Computational chemistry uses computer simulations and mathematical models to explore molecules, reactions, and materials at the atomic level. It helps you understand molecular behavior, stability, and interactions through techniques like molecular modeling, energy minimization, and quantum mechanics. Software tools and high-performance computing enable efficient exploration of complex systems. Despite current challenges like accuracy limits and hardware constraints, ongoing advancements improve capabilities. Continue exploring to uncover how these innovations shape the future of science and research.
Key Takeaways
- Computational chemistry uses computer simulations and mathematical models to study molecular structures, reactions, and interactions.
- Techniques include molecular modeling, energy minimization, quantum mechanics, and molecular dynamics.
- It aids in drug discovery, material design, and understanding molecular behavior, often supported by advanced software tools.
- Current challenges involve hardware limitations, accuracy of models, and scalability for complex systems.
- Future trends focus on integrating AI, enhancing efficiency, and developing sustainable, cost-effective simulation methods.
What Is Computational Chemistry?
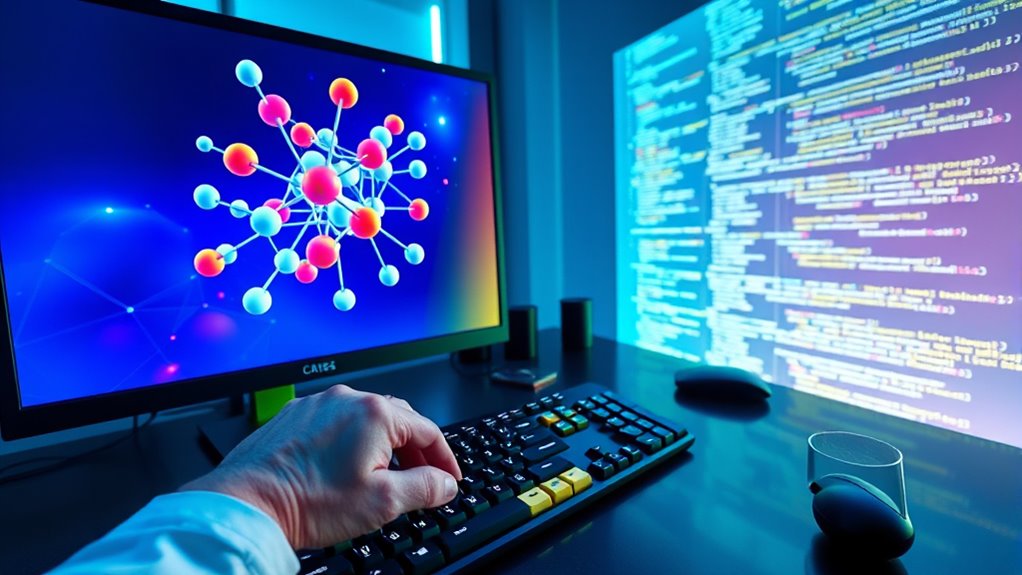
Computational chemistry is a branch of chemistry that uses computer simulations and mathematical models to understand and predict the behavior of molecules and chemical systems. At its core, it involves techniques like molecular modeling, where you create virtual representations of molecules to study their structure and interactions. Energy minimization is a key process in this field; it helps you find the most stable molecular conformations by reducing a molecule’s potential energy. By applying algorithms to optimize molecular geometries, you can explore how molecules behave, react, and interact with each other. This approach allows you to gain insights that are often difficult or impossible to observe experimentally, making computational chemistry a powerful tool for advancing chemical research and discovery. Additionally, advances in computational methods continue to expand the possibilities for simulating complex chemical phenomena, offering new ways to understand and manipulate chemical systems. These innovations are increasingly supported by high-performance computing, enabling more detailed and accurate simulations than ever before. The integration of machine learning techniques is also beginning to revolutionize how predictions are made within this field, enhancing the efficiency and scope of simulations. Furthermore, ongoing research into AI security helps ensure that these powerful tools are used responsibly and safely in scientific advancements.
Key Techniques and Methods in Computational Chemistry
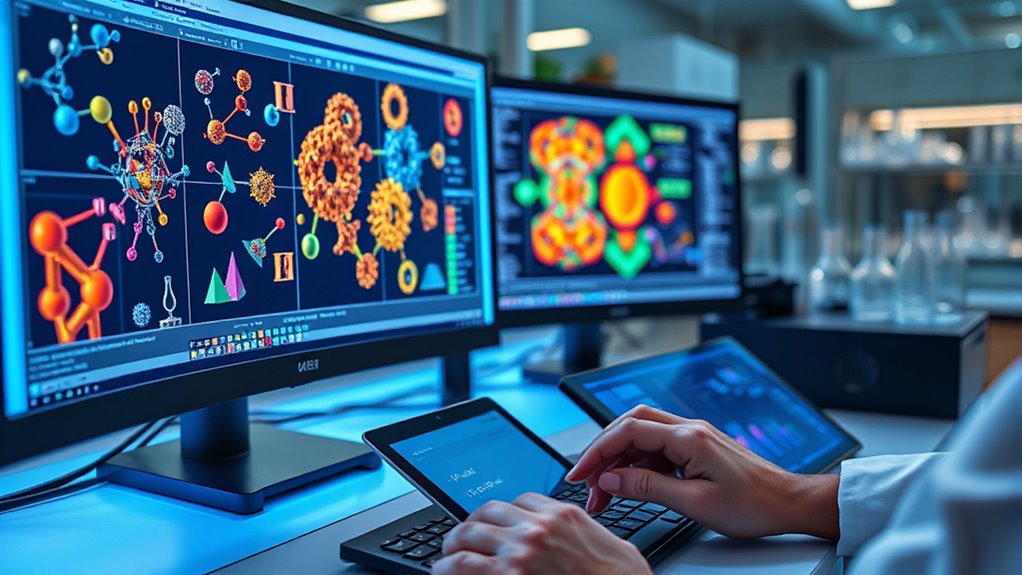
To effectively explore molecular behavior and reactions, chemists rely on a variety of key techniques and methods in computational chemistry. Molecular docking helps you predict how molecules, like drugs and proteins, fit together, revealing potential binding sites and affinities. Conformational analysis allows you to examine different shapes a molecule can adopt, helping you identify the most stable structures. These methods enable you to simulate interactions and understand molecular flexibility without physical experiments. You can optimize molecular geometries, study energy landscapes, and predict reactivity. By combining docking and conformational analysis, you gain detailed insights into how molecules behave in various environments. Additionally, understanding color accuracy can be essential when considering legal implications of molecular interactions in certain cases. Incorporating automation technology can further enhance modeling efficiency and accuracy in computational studies. Implementing data analysis tools can help interpret complex simulation results more effectively. These techniques form the foundation for more advanced modeling and are essential tools in your computational chemistry toolkit.
Applications in Drug Discovery and Material Design
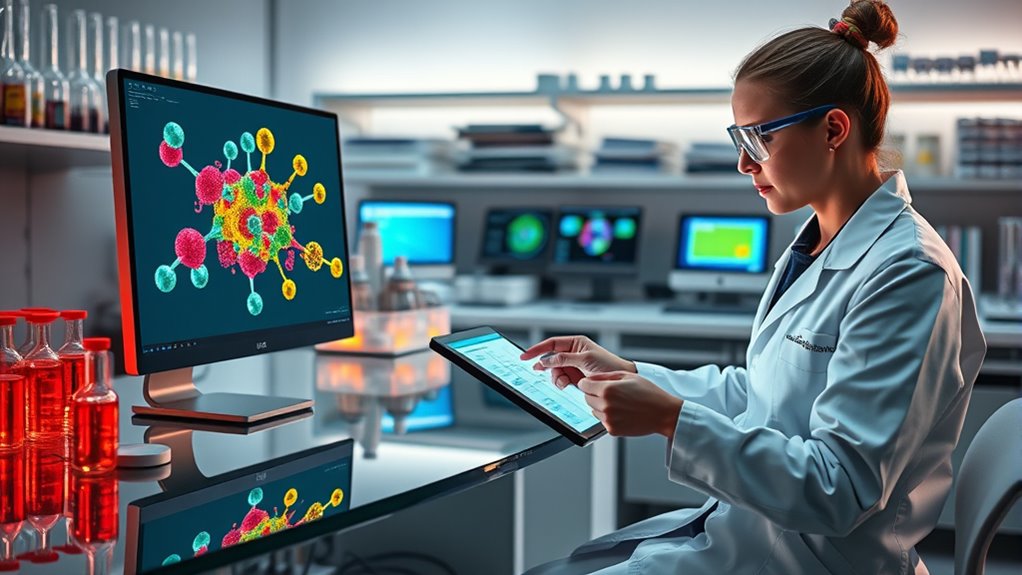
The techniques you’ve learned, like molecular docking and conformational analysis, play a vital role in advancing drug discovery and material design. Molecular docking helps you predict how a drug candidate fits into a target protein’s active site, speeding up the screening process. Pharmacophore modeling allows you to identify key features necessary for biological activity, guiding the design of new compounds. Visualize a virtual library of molecules, each tested against a target, revealing ideal fits. Imagine tailoring molecules to match specific binding pockets or key pharmacophore features, reducing costly lab experiments. These computational methods streamline development, improve accuracy, and enable rapid innovation in pharmaceuticals and advanced materials. Your ability to harness these tools accelerates discovery, making it more efficient and targeted. Financial aspects can also influence project budgets and resource allocation in research and development. Additionally, understanding offensive security techniques enhances the accuracy of computational predictions by simulating potential vulnerabilities, leading to stronger and more reliable designs.
The Role of Quantum Mechanics and Molecular Mechanics
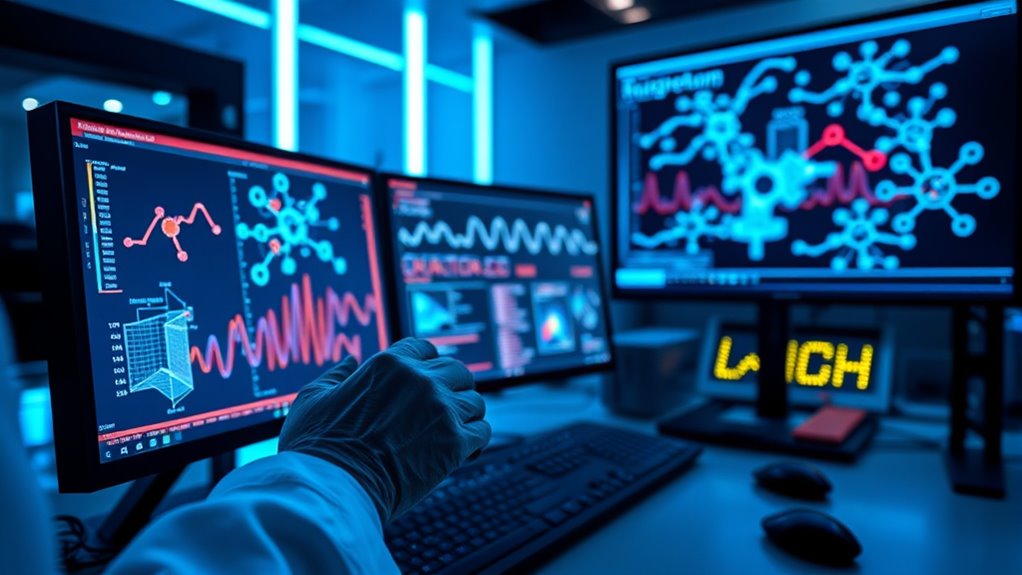
Since molecules exhibit both quantum and classical behaviors, understanding their interactions requires different computational approaches. Quantum mechanics models phenomena like quantum tunneling and the formation of molecular orbitals, which are essential for accurate predictions of reactivity and bonding. Molecular mechanics simplifies molecules as classical systems, focusing on bond lengths and angles to optimize structures efficiently. Additionally, UV radiation exposure can influence molecular interactions, highlighting the importance of considering environmental factors in computational models. Recognizing the role of residency requirements in legal processes can also inform the development of more precise simulation methods. Incorporating Honda Tuning principles, such as performance optimization techniques, can also inform the development of more precise simulation methods. Recognizing the role of emotional support in scientific research can improve team collaboration and overall project success.
Software Tools and Computational Resources
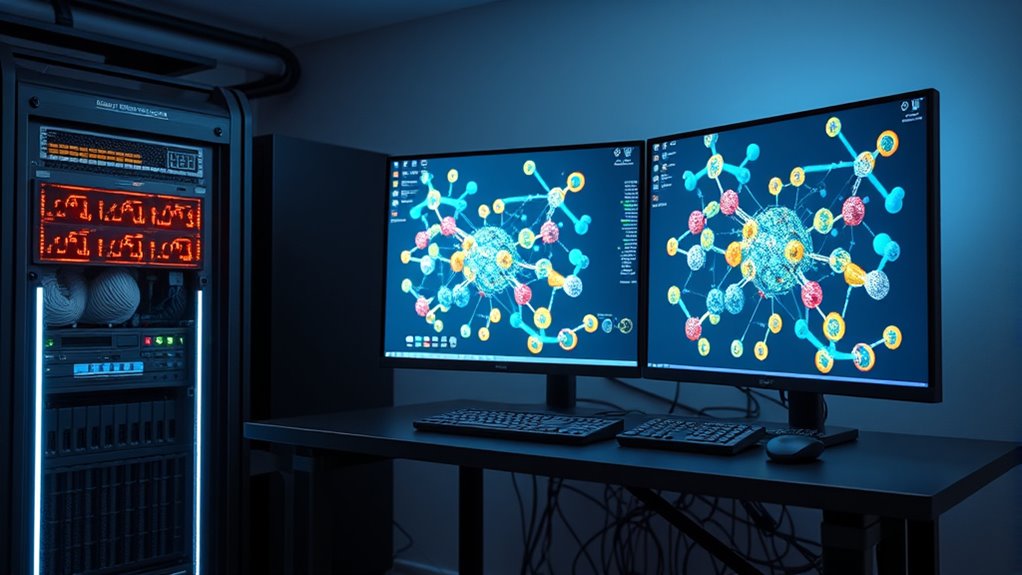
Advancements in computational chemistry rely heavily on specialized software tools designed to simulate molecular behavior accurately. These tools feature intuitive software interfaces that make complex calculations accessible, even for non-experts. You’ll find a range of options, from open-source programs to commercial packages, each requiring proper computational licensing. These licenses often dictate usage limits and features, shaping your workflow. Popular software tools include programs for quantum calculations, molecular dynamics, and visualization. Imagine:
- A sleek interface guiding your input and analysis
- Licensing options offering flexibility or strict access
- Visual representations transforming data into understandable images
- Cloud-based resources enabling large-scale computations
- Regular updates and maintenance enhance software performance and reliability. Incorporating user-friendly features can improve the overall experience and efficiency of your computational tasks. Additionally, choosing software with comprehensive documentation can help users troubleshoot and optimize their workflows effectively. Recognizing the importance of software versatility can also expand your research capabilities and adapt to various project needs.
Challenges and Limitations of Computational Approaches
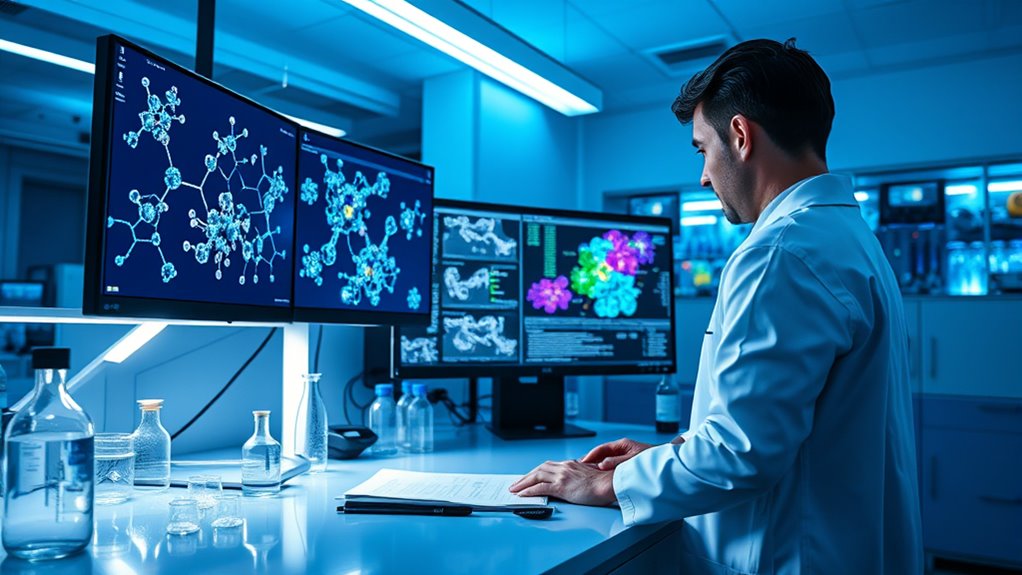
You might find that limited computational power can slow down complex simulations or restrict the size of systems you can study. Additionally, the accuracy of predictions often depends on the methods and approximations used, which can introduce errors. Recognizing these challenges helps you understand the current boundaries of computational chemistry. For example, the refrigeration cycle principles underpin many modern heating and cooling systems, illustrating how energy transfer processes are fundamental across various scientific and engineering disciplines. Moreover, ongoing advancements in computational algorithms aim to address these limitations and expand the capabilities of simulations. As a result, improving simulation efficiency is a key focus within the field to enable more complex and realistic models. Limitations in computational resources can also impede large-scale or highly detailed simulations, emphasizing the need for continued technological progress and algorithmic development.
Computational Power Constraints
Computational power constraints considerably impact the scope and accuracy of simulations in computational chemistry. You often face hardware limitations that restrict the size and complexity of models you can run. Scalability challenges mean that as molecules grow larger or simulations become more detailed, your computing resources may fall short. This can lead to longer processing times or simplified models that miss critical details. Visualize:
- A supercomputer struggling under massive data loads
- Limited memory bottlenecking detailed simulations
- Processing units overheating during intense calculations
- Software unable to efficiently distribute tasks across multiple cores
These issues highlight how hardware limitations and scalability challenges shape what you can achieve. Overcoming these constraints requires balancing model complexity with available computational power, often forcing compromises in simulation depth or speed.
Accuracy of Predictions
Achieving accurate predictions in computational chemistry is inherently challenging because models rely on approximations that can introduce errors. These limitations affect the predictive accuracy of simulations, making it difficult to perfectly match experimental results. Factors like choice of method, basis sets, and potential energy surfaces influence outcomes, and small variations can lead to significant discrepancies. To improve confidence, you should compare computational results with experimental validation whenever possible. Recognizing these constraints helps you interpret predictions more critically and avoid overconfidence in your models. While ongoing developments aim to enhance accuracy, understanding current limitations ensures you use computational tools effectively, properly balancing theoretical insights with experimental data to make informed decisions.
Future Trends and Emerging Developments
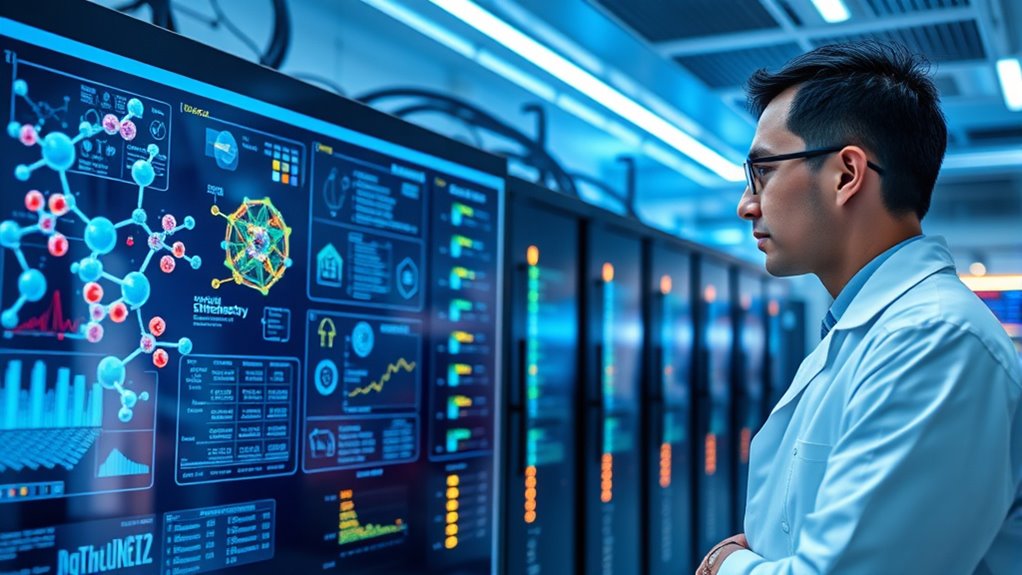
As technology advances, the field of computational chemistry is poised to undergo significant transformations. You’ll see increased AI integration, enabling faster, more accurate simulations and predictions. Sustainability efforts will drive the development of eco-friendly models that minimize resource use. Emerging tools will enhance your ability to design novel materials and pharmaceuticals efficiently. Imagine complex molecular interactions visualized in real-time, powered by machine learning algorithms. You might also leverage cloud computing for collaborative research, accelerating discoveries across borders. These innovations will make your research more sustainable, cost-effective, and impactful.
- Real-time molecular visualization with AI
- Eco-conscious simulation models
- Cloud-based collaborative platforms
- Rapid drug and material discovery
Frequently Asked Questions
How Accurate Are Computational Chemistry Predictions Compared to Experimental Results?
You might wonder about the accuracy of computational chemistry predictions compared to experiments. Generally, the theoretical precision varies depending on the methods used, but many predictions show strong predictive reliability. Advanced techniques can closely match experimental results, often within a few kcal/mol for energies or tenths of a nanometer for structures. While not perfect, computational chemistry provides valuable insights that complement experimental data, making it a useful tool in research.
What Are the Ethical Considerations in Using Computational Chemistry?
Imagine a digital vault where your data privacy feels protected, yet ethical questions about computational chemistry still arise. You must consider how using sensitive data responsibly and respecting intellectual property rights safeguard trust. As you navigate this landscape, guarantee transparency in algorithms and data handling, preventing misuse. Balancing innovation with ethics, you help protect both scientific progress and the rights of creators, fostering a responsible, trustworthy scientific community.
How Accessible Is Computational Chemistry for Beginners and Students?
Computational chemistry is increasingly accessible for beginners and students thanks to user-friendly software accessibility and online resources. While there’s a learning curve, many programs offer tutorials and free versions that simplify the process. You can start exploring concepts without advanced skills, gradually building your knowledge. With dedication and practice, you’ll find it easier to understand and apply computational tools, making this field more approachable than ever.
Can Computational Methods Replace Laboratory Experiments Entirely?
You wonder if computational methods can fully replace laboratory experiments. While simulated reactions and virtual labs provide valuable insights and reduce costs, they can’t replicate all real-world variables. Hands-on experiments teach you practical skills, observe unpredictable outcomes, and develop intuition. So, computational approaches complement lab work but won’t entirely replace the experience and understanding gained from actual laboratory experiments.
What Are the Environmental Impacts of Large-Scale Computational Simulations?
When you consider the environmental impacts of large-scale computational simulations, you should think about energy consumption and data privacy. These simulations require significant energy, often from non-renewable sources, which contributes to carbon emissions. Additionally, handling vast amounts of data raises concerns about data privacy and security. While they reduce the need for physical experiments, it is crucial to optimize computational efficiency and adopt sustainable practices to minimize environmental footprints.
Conclusion
As you explore computational chemistry, remember it’s like opening a treasure chest of molecular secrets, revealing insights that were once hidden. With your understanding of key techniques, applications, and emerging trends, you’ll be equipped to explore new frontiers in science. Embrace the challenges as stepping stones, and let the evolving software tools be your compass. The journey ahead is an exciting voyage into the tiny universe within molecules, waiting for you to discover its wonders.